AlexNet을 Pytorch를 활용하여 구현하고자 한다.
https://arsetstudium.tistory.com/18에서 공부한 내용을 토대로 구현보면 아래와 같다.
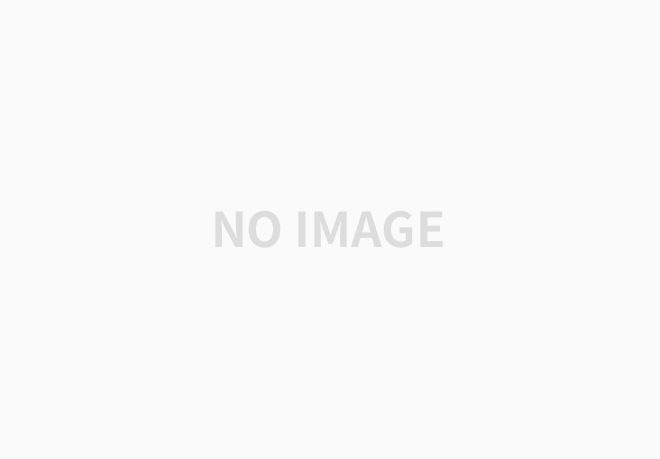
첫 번째 구현
class AlexNet(nn.Module):
def __init__(self, num_classes = 1000, dropout = 0.5):
super().__init__()
self.model = nn.Sequential(
# Extracting Fetures Part
# First Convolution
nn.Conv2d(3, 96, kernel_size = 11, stride = 4, padding = 2),
# Activation function is applied
nn.ReLU(),
# Max pooling
nn.MaxPool2d(kernel_size = 3, stride = 2),
# Second Convolution
nn.Conv2d(96, 256, kernel_size = 5, padding = 2),
# Activation
nn.ReLU(),
# Max pooling
nn.MaxPool2d(kernel_size = 3, stride = 2),
nn.Conv2d(256, 384, kernel_size = 3, padding = 1),
nn.ReLU(),
nn.Conv2d(384, 384, kernel_size = 3, padding = 1),
nn.ReLU(),
nn.Conv2d(384, 256, kernel_size = 3, padding = 1),
nn.MaxPool2d(kernel_size = 6, stride = 2),
"""여기까지는 앞과 같은 연산이다.
이때, Conv2d는 우리가 흔히 아는 matrix 상에서 상하좌우로,
x축과 y축으로 움직이는 컨볼루션을 의미한다.
"""
# Flatten은 FC layer, MLP를 적용하기 위해
# features를 1차원 벡터로 변경하는 작업이다.
nn.Flatten(start_dim = 1),
# First FC layer
nn.Linear(256 * 5 * 5, 4096),
# First dropout
nn.Dropout(p=dropout),
# Second FC layer
nn.Linear(4096, 4096),
# Second dropout
nn.Dropout(p=dropout),
# Final FC layer
nn.Linear(4096, num_classes),
# Return probability by softmax function
nn.Softmax()
)
def forward(self, x):
y = self.model(x)
return y
가장 기본적으로 생각할 수 있는 구현으로 피쳐를 학습하는 부분과 분류하는 부분을 모두 하나의 nn.Sequential로 묶는 방법이다.
AlexNet을 구성하는 각 layer의 의미는 위 코드에 붙여 넣었다.
여기서 처음 PyTorch를 접할 때 주의해야할 점은 Conv2d, MaxPool2d, Linear 정도라고 생각한다.
Conv와 MaxPool에 2d가 붙는 이유는, 우리가 흔히 아는 matrix 상에서 상하좌우로 움직이는,
즉 x축과 y축으로 움직이는 window를 이용한 컨볼루션이나 맥스 풀링을 의미하기 때문이다.
1d convolution이 있나 생각할 수 있는데, Convolutional Neural Networks for Sentence Classification, Yoon Kim, EMNLP 2014 같은 논문을 보면 1d Convolution으로, y축으로만 움직임을 확인할 수 있다.
또한 Conv2d의 자세한 arguments는 다음과 같다.
Conv2d(in_channels, out_channels, kernel_size,
stride=1, padding=0, dilation=1, groups=1, bias=True,
padding_mode='zeros', device=None, dtype=None)
첫 번째 Conv2d을 이용하여 코드의 자세한 내용을 해석하면 다음과 같다.
Conv2d(3, 96, kernel_size = 11, stride=4, padding=2)
우선 3은 in_channels로 들어오는 채널 = depth = filters의 숫자이며,
out_channels는 output으로 나가는 채널 = depth = filters의 숫자이며,
kernel_size는 기본적으로 정사각형을 사용하므로 window = kernerl의 사이즈는 11 x 11이 된다.
stride와 padding은 우리가 아는 스트라이드와 패딩을 얼마만큼 할지를 결정하며,
기본적인 padding_mode = 패딩의 방법은 zero padding이다.
또한 bias=True라 디폴트 옵션이기 때문에 bias term은 기본적으로 사용한다.
Linear는 간단한 Affine Transformation이다.
nn.Linear(in_features, out_features, bias=True, device=None, dtype=None)
따라서, nn.Linear(4096, 1000)이라면
y = xA + b의 연산에서, x가 4096-dim 벡터고, matrix A가 4096 x 1000이고, 벡터 b가 1000-dim
을 나타내고 이를 수행함을 알 수 있다.
batch size 5개의 256 x 256 RGB 이미지를 넣는 상황을 가정하면 다음과 같은 결과를 얻을 수 있다.
# random image tensor
a = torch.rand(5, 3, 256, 256)
a.shape
>> torch.Size([5, 3, 256, 256])
# declare model
model = AlexNet()
b = model(a)
b.shape
>> torch.Size([5, 1000])
class가 1000개 일 때, 5개의 이미지에 대해서 1000개의 라벨에 대한 예측값을 리턴함을 알 수 있다.
두 번째 구현
이번에는 다른 구현 방법을 살펴보자.
class AlexNet(nn.Module):
def __init__(self, num_classes = 1000, dropout = 0.5):
super().__init__()
self.features = nn.Sequential(
# Extracting Fetures Part
nn.Conv2d(3, 96, kernel_size = 11, stride = 4, padding = 2),
nn.ReLU(),
nn.MaxPool2d(kernel_size = 3, stride = 2),
nn.Conv2d(96, 256, kernel_size = 5, padding = 2),
nn.ReLU(),
nn.MaxPool2d(kernel_size = 3, stride = 2),
nn.Conv2d(256, 384, kernel_size = 3, padding = 1),
nn.ReLU(),
nn.Conv2d(384, 384, kernel_size = 3, padding = 1),
nn.ReLU(),
nn.Conv2d(384, 256, kernel_size = 3, padding = 1),
nn.MaxPool2d(kernel_size = 6, stride = 2)
)
self.classifier = nn.Sequential(
# Classification Part
nn.Linear(256 * 5 * 5, 4096),
nn.Dropout(p=dropout),
nn.Linear(4096, 4096),
nn.Dropout(p=dropout),
nn.Linear(4096, num_classes),
nn.Softmax()
)
def forward(self, x):
y = self.features(x)
y = torch.flatten(y, start_dim = 1)
y = self.classifier(y)
return y
위 코드를 보면 피쳐를 학습하는 convolutional layers와 max pooling layers를 features로,
fc layers가 있는 부분을 classifier로 구분했다.
이는 학습시킨 AlexNet을 다른 이미지에 대한 pre-trained 모델로 사용할 경우 classifier만 바꿔서 학습하면 편하기 때문이다.
# decalre model
model = AlexNet()
# forward
c = model.forward(a)
c
>> torch.Size([5, 1000])
이번에도 5개의 이미지에 대해서 1000개의 라벨에 대한 예측값을 리턴함을 알 수 있다.
Flatten tools in PyTorch
Flatten은 다차원의 텐서를 1-d 텐서로 변형하는 연산인데 위에서도 알 수 있듯이 다양한 방법이 있다.
pytorch에서는 torch.Tensor.flatten, torch.flatten, nn.Flatten의 총 세 가지가 방법이 있다.
자세한 설명은 아래와 같다.
Flattening is available in three forms in PyTorch
- As a tensor method (oop style) torch.Tensor.flatten applied directly on a tensor: x.flatten().
- As a function (functional form) torch.flatten applied as: torch.flatten(x).
- As a module (layer nn.Module) nn.Flatten(). Generally used in a model definition.
All three are identical and share the same implementation, the only difference being nn.Flatten has start_dim set to 1 by default to avoid flattening the first axis (usually the batch axis). While the other two flatten from axis=0 to axis=-1 - i.e. the entire tensor - if no arguments are given.
출처: https://stackoverflow.com/questions/65993494/difference-between-torch-flatten-and-nn-flatten
Difference between torch.flatten() and nn.Flatten()
What are the differences between torch.flatten() and torch.nn.Flatten()?
stackoverflow.com
AlexNet from torchvision
이번에는 torchvision에서 제공하는 AlexNet 코드를 통해 구현한 코드를 비교하고자 한다.
import torchvision.models as models
alexnet = models.alexnet(pretrained=True)
alexnet
>>
AlexNet(
(features): Sequential(
(0): Conv2d(3, 64, kernel_size=(11, 11), stride=(4, 4), padding=(2, 2))
(1): ReLU(inplace=True)
(2): MaxPool2d(kernel_size=3, stride=2, padding=0, dilation=1, ceil_mode=False)
(3): Conv2d(64, 192, kernel_size=(5, 5), stride=(1, 1), padding=(2, 2))
(4): ReLU(inplace=True)
(5): MaxPool2d(kernel_size=3, stride=2, padding=0, dilation=1, ceil_mode=False)
(6): Conv2d(192, 384, kernel_size=(3, 3), stride=(1, 1), padding=(1, 1))
(7): ReLU(inplace=True)
(8): Conv2d(384, 256, kernel_size=(3, 3), stride=(1, 1), padding=(1, 1))
(9): ReLU(inplace=True)
(10): Conv2d(256, 256, kernel_size=(3, 3), stride=(1, 1), padding=(1, 1))
(11): ReLU(inplace=True)
(12): MaxPool2d(kernel_size=3, stride=2, padding=0, dilation=1, ceil_mode=False)
)
(avgpool): AdaptiveAvgPool2d(output_size=(6, 6))
(classifier): Sequential(
(0): Dropout(p=0.5, inplace=False)
(1): Linear(in_features=9216, out_features=4096, bias=True)
(2): ReLU(inplace=True)
(3): Dropout(p=0.5, inplace=False)
(4): Linear(in_features=4096, out_features=4096, bias=True)
(5): ReLU(inplace=True)
(6): Linear(in_features=4096, out_features=1000, bias=True)
)
)
aa = alexnet(a)
aa.shape
>> torch.Size([5, 1000])
컨볼루션 레이어들의 필터 수가 다름을 발견할 수 있다.
이에 대한 답변을 아래의 링크들에서 확인할 수 있었다.
https://github.com/pytorch/vision/pull/463#issuecomment-379214941
https://github.com/akrizhevsky/cuda-convnet2/blob/master/layers/layers-imagenet-1gpu.cfg#L81
Figure 1에 나온 파라미터들은 멀티 GPU를 사용할 때의 필터 수이고,
싱글 GPU일 때는 torchvision에 구현된 필터의 수를 사용했다고 한다.
AlexNet Model code from torchvision github
보다 자세한 내용을 알아보기 위해서 아래 링크의 github 구현 코드를 살펴보고자 한다.
https://github.com/pytorch/vision/blob/main/torchvision/models/alexnet.py
class AlexNet(nn.Module):
def __init__(self, num_classes: int = 1000, dropout: float = 0.5) -> None:
super().__init__()
_log_api_usage_once(self)
self.features = nn.Sequential(
nn.Conv2d(3, 64, kernel_size=11, stride=4, padding=2),
nn.ReLU(inplace=True),
nn.MaxPool2d(kernel_size=3, stride=2),
nn.Conv2d(64, 192, kernel_size=5, padding=2),
nn.ReLU(inplace=True),
nn.MaxPool2d(kernel_size=3, stride=2),
nn.Conv2d(192, 384, kernel_size=3, padding=1),
nn.ReLU(inplace=True),
nn.Conv2d(384, 256, kernel_size=3, padding=1),
nn.ReLU(inplace=True),
nn.Conv2d(256, 256, kernel_size=3, padding=1),
nn.ReLU(inplace=True),
nn.MaxPool2d(kernel_size=3, stride=2),
)
self.avgpool = nn.AdaptiveAvgPool2d((6, 6))
self.classifier = nn.Sequential(
nn.Dropout(p=dropout),
nn.Linear(256 * 6 * 6, 4096),
nn.ReLU(inplace=True),
nn.Dropout(p=dropout),
nn.Linear(4096, 4096),
nn.ReLU(inplace=True),
nn.Linear(4096, num_classes),
)
def forward(self, x: torch.Tensor) -> torch.Tensor:
x = self.features(x)
x = self.avgpool(x)
x = torch.flatten(x, 1)
x = self.classifier(x)
return x
필터의 수 외에도 ReLU에서 inplace=True가 다른 것을 알 수 있다.
inplace=True 조건을 준다면, ReLU로 들어온 텐서 자체의 값이 바뀐다고 한다.
이를 통해서 메모리를 아낄 수 있는데, 원본 값을 사라지게 하기 때문에 그라디언트 계산에서 문제가 생길 수 있다고 한다.
Apdative Average Pooling
nn.AdaptiveAvgPool2d는 처음 보는 항목인데 N, C, H, W의 크기의 데이터를 원하는 사이즈인 N, C, S, T로 변환해준다.
이때의 변환은 이름에서 알 수 있듯이 평균, average다.
m = nn.AdaptiveAvgPool2d((5, 7))
a = torch.rand(1, 64, 87, 9)
print(m(a).shape)
>> torch.Size([1, 64, 5, 7])
위 AlexNet 코드에서는 W와 H의 size를 6x6으로 픽스해서, 첫번째 FC의 in_features인 256 x 6x 6의 크기를 맞춰주는 역할을 한다.
자세한 내용은 다음 URL에 나와있다.
https://pytorch.org/docs/stable/generated/torch.nn.AdaptiveAvgPool2d.html
실제 학습 코드
이번에는 구체적인 학습이다. Loss는 Classificiation이기 때문에 cross entropy를,
classification의 성능을 알아보기 위해서 accuracy와 F1 macro와 F1 micro를 살펴보았다.
# Declare Model
model = AlexNet(num_classes = 10)
# Initialze Parameters
# model.apply(init_weights)
# Define Loss function
# Default reduction option is mean
cross_entropy = nn.CrossEntropyLoss()
# Define Optimizer
optimizer = torch.optim.SGD(model.parameters(), lr = 0.01,
momentum = 0.9, weight_decay = 0.0005)
model.to(device)
start = time.time()
for epoch in range(config.n_epochs):
epoch_loss = 0.0
preds_list = []
labels_list = []
for images, labels in train_loader:
# Clear gradients
optimizer.zero_grad()
# Predict Labels
predictions = model(images)
loss = cross_entropy(predictions, labels)
# Backpropogation
loss.backward()
# Next Step
optimizer.step()
epoch_loss += loss.item()
# Extract indices having max probs and filter out the rest
preds_list.extend(predictions.argmax(dim=1).detach().cpu().numpy())
labels_list.extend(labels.detach().cpu().numpy())
train_acc = accuracy_score(labels_list, preds_list)
train_f1_macro = f1_score(labels_list, preds_list, average = 'macro')
train_f1_micro = f1_score(labels_list, preds_list, average = 'micro')
print("Epoch {0} - Train CE loss: {1}, ACC: {2}, Macro F1: {3}".format(
epoch, epoch_loss, train_acc, train_f1_macro))
print("Micro F1: ", train_f1_micro)
학습하고자 하는 NN 모델을 선언하고, optimizer를 설정하고 dataloader를 활용하여
미니배치 학습을 시키는 작업을 위의 코드를 통해 수행할 수 있다.
Cross Entropy에서 reduction option을 통해 하나의 batch에 loss를 구한 다음
sum으로 리턴할건지 mean으로 리턴할건지를 결정한다.
sum은 절대적인 크기기 때문에 batch size 자체에 영향을 받기도 하고,
batch의 모든 개체의 영향력을 보기 위해서는 mean을 설정하는 것이 낫다.
이때 dataloader의 last_drop=False라면 마지막 배치는 batch_size와 숫자가 다를 수 있기 때문에
epoch loss를 구할 때 주의해야 한다.
하지만 train뿐만 아니라 validation도 수행해야 하므로 중복되는 코드가 많아진다.
따라서 train을 위한 코드와 validation을 위한 코드를 별개로 만들어서 코드를 보다 간결하게 만들고자 한다.
from sklearn.metrics import accuracy_score, f1_score
def train_batches(loader, model, optimizer, loss_function):
# Set the model to train
model.train()
epoch_loss = 0.0
preds_list = []
labels_list = []
for images, labels in loader:
# Clear gradients
optimizer.zero_grad()
# Move to device
images = images.to(device)
labels = labels.to(device)
# Predict Labels
predictions = model(images)
loss = loss_function(predictions, labels)
# Backpropogation
loss.backward()
# Next Step
optimizer.step()
epoch_loss += loss.item()
# Extract indices having max probs and filter out the rest
preds_list.extend(predictions.argmax(dim=1).detach().cpu().numpy())
labels_list.extend(labels.detach().cpu().numpy())
train_acc = accuracy_score(labels_list, preds_list)
train_f1_macro = f1_score(labels_list, preds_list, average = 'macro')
train_f1_micro = f1_score(labels_list, preds_list, average = 'micro')
train_acc = round(train_acc, 4)
train_f1_macro = round(train_f1_macro, 4)
train_f1_micro = round(train_f1_micro, 4)
print("Epoch {0} - Train Data, CE loss: {1}, ACC: {2}, Macro F1: {3}".format(
epoch, epoch_loss, train_acc, train_f1_macro))
print("Micro F1: ", train_f1_micro)
def test_batches(loader, model, loss_function, process_name = 'Test'):
# Set the model to evaluate
model.eval()
epoch_loss = 0.0
preds_list = []
labels_list = []
for images, labels in loader:
# Move to device
images = images.to(device)
labels = labels.to(device)
# Predict Labels
predictions = model(images)
loss = loss_function(predictions, labels)
epoch_loss += loss.item()
# Extract indices having max probs and filter out the rest
preds_list.extend(predictions.argmax(dim=1).detach().cpu().numpy())
labels_list.extend(labels.detach().cpu().numpy())
acc = accuracy_score(labels_list, preds_list)
f1_macro = f1_score(labels_list, preds_list, average = 'macro')
f1_micro = f1_score(labels_list, preds_list, average = 'micro')
acc = round(acc, 4)
f1_macro = round(f1_macro, 4)
f1_micro = round(f1_micro, 4)
print("Epoch {0} - {4} Data, CE loss: {1}, ACC: {2}, Macro F1: {3}".format(
epoch, epoch_loss, acc, f1_macro, process_name))
print("Micro F1: ", f1_micro)
return epoch_loss
# Declare Model
model = AlexNet(num_classes = 10)
# Initialze Parameters
# model.apply(init_weights)
# Define Loss function
cross_entropy = nn.CrossEntropyLoss()
# Define Optimizer
optimizer = torch.optim.SGD(model.parameters(), lr = 0.01,
momentum = 0.9, weight_decay = 0.0005)
model.to(device)
start = time.time()
for epoch in range(config.n_epochs):
epoch_start = time.time()
# Train Part
print('='*20,f" Epoch {epoch}: Train Step ",'='*20)
train_batches(train_loader, model, optimizer, cross_entropy)
end = time.time()
duration = end - epoch_start
print(f"Train Time: {duration: .4f}")
# Validation Part
print('='*20,f" Epoch {epoch}: Validation Step ",'='*20)
val_start = time.time()
val_loss = test_batches(val_loader, model, cross_entropy, process_name = 'Validation')
end = time.time()
duration = end - val_start
print(f"Validation Time: {duration: .4f}")
duration = end - epoch_start
print(f"Taken Time for Epoch {epoch} is {duration: .4f}")
Transfer Learning
PyTorch의 AlexNet pretrained model로 CIFAR-10을 예측했다.
Pre-trained model을 가져와서 Classifier를 변경하는 transfer learning 코드는 다음과 같다.
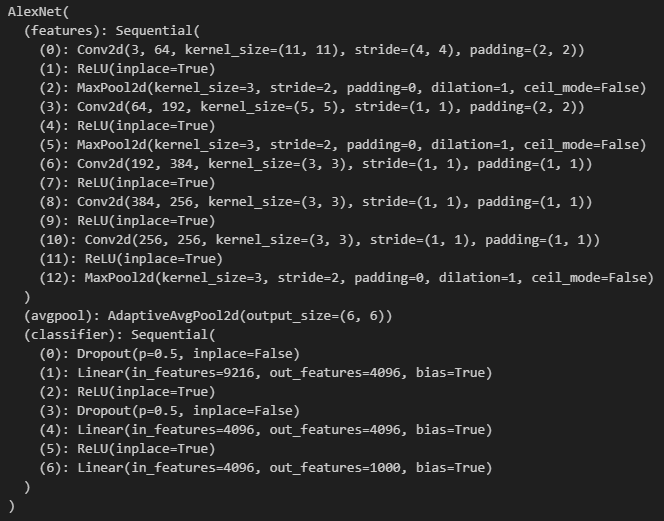
# Classifier의 6번째 항목을 바꿔준다.
# Class의 개수를 1000개에서 10개로 변경
last_d_features = model.classifier[6].in_features
# From 1000 to 10
model.classifier[6] = nn.Linear(last_d_features, 10)
model
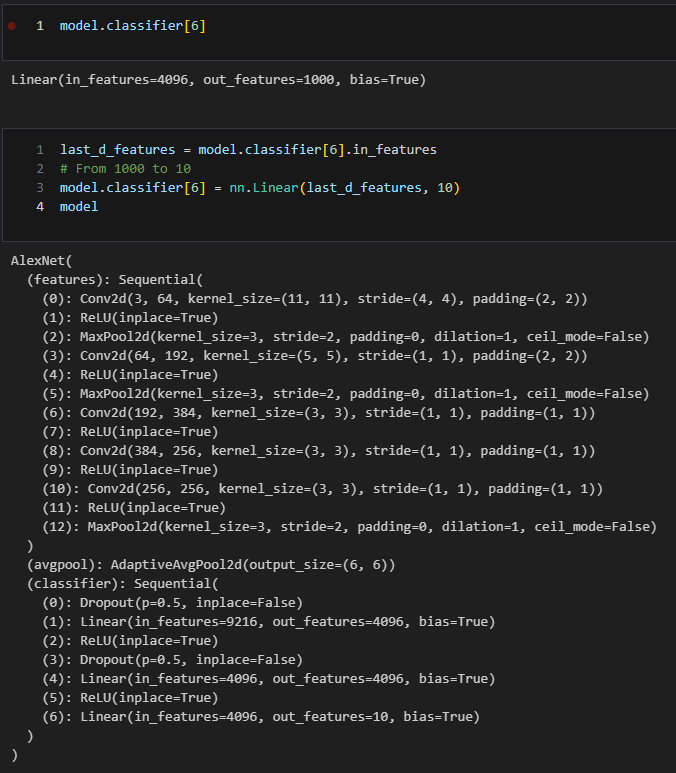
결과는 다음과 같다.
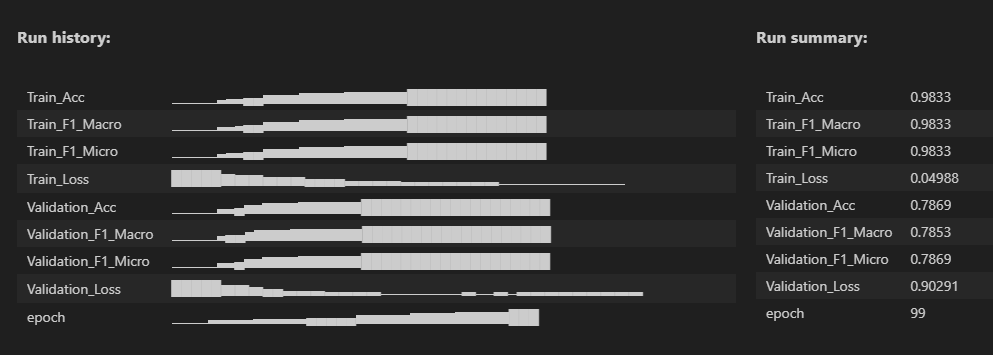
Figure 4는 wandb를 통한 성능에 대한 요약으로, train accuracy, F1 macro와 micro는 0.98이지만 validation은 0.78정도로 낮게 나온 것을 알 수 있다.
Confusion Matrix Display
Class별 accuracy를 살펴보기 위해서 Scikit-Learn의 ConfusionMatrixDisplay를 사용한다.
from sklearn.metrics import ConfusionMatrixDisplay
import matplotlib.pyplot as plt
classes = [
"airplane",
"automobile",
"bird",
"cat",
"deer",
"dog",
"frog",
"horse",
"ship",
"truck",
]
disp = ConfusionMatrixDisplay.from_predictions(
y_train,
preds_list,
display_labels=classes
)
fig = disp.figure_
fig.set_figwidth(10)
fig.set_figheight(10)
fig.suptitle('Plot of confusion matrix')
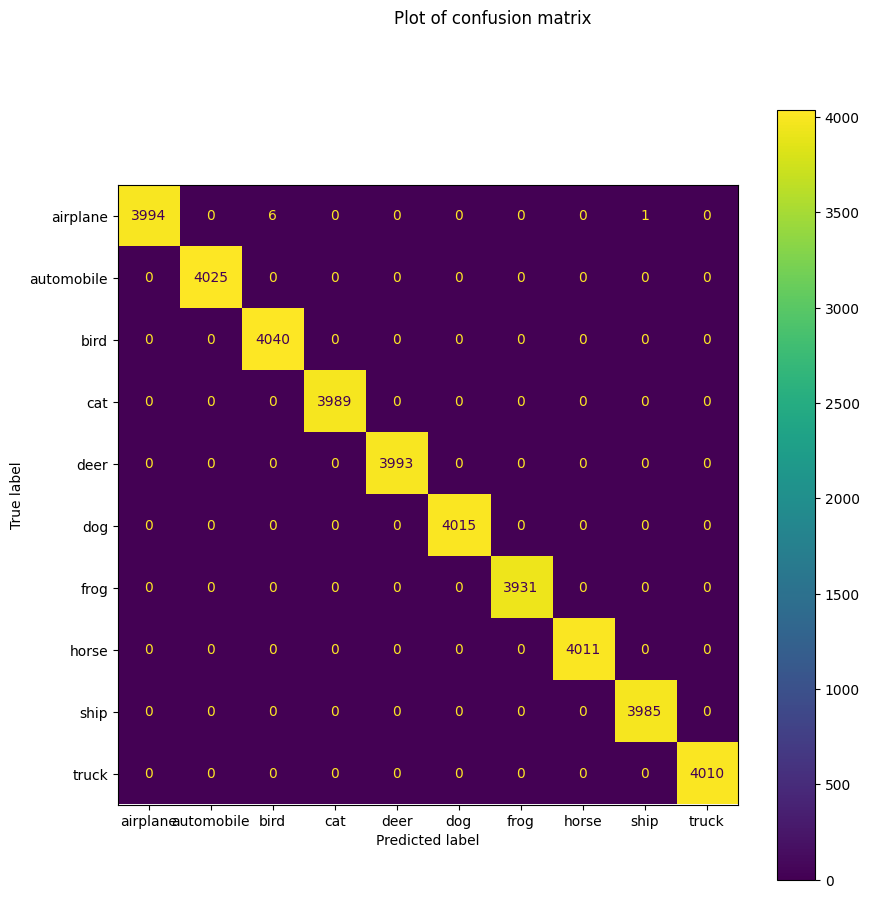
Training Data에 대한 confusion matrix인데 모든 클래스에 대해 잘 학습했음을 확인할 수 있다.
본래는 train 데이터는 성능이 높게 나오지만 validation에 대해서 성능이 낮은 경우,
일반화 성능이 낮다고 판단되어 data augmentation을 하거나 기타 오버피팅을 방지하기 위한 조치를 취한다.
하지만 앞으로 VGG, ResNet, ViT 등등 AlexNet보다 성능이 좋은, 보다 최신의 모델이 많으므로 이는 차후에 다른 포스트를 통해서 적고자 한다.
'Computer Vision' 카테고리의 다른 글
GoogLeNet = Inception v1 (2014) 논문 리뷰 (0) | 2024.04.03 |
---|---|
VGGNet PyTorch Code Implementation (0) | 2024.04.02 |
VGGNet (2014) 논문 리뷰 (0) | 2024.04.01 |
AlexNet (2012) 논문 리뷰 (0) | 2024.02.28 |
Computer Vision 소개 (0) | 2024.02.21 |